Is Winter Coming? (2024)

Is Winter Coming?
Thoughts on articifial intelligence and lofty expectations.
Spring 2024
In the 1960s, AI researchers tried - and failed - to deliver machine translation of Russian to English. Overly confident researchers, lazy journalists and far too optimistic tech utopianists all built expectations that couldn't be met. Eventually, the lack of results put a stop to the previously generous funding. The project was halted and interest in AI faltered. This was the first "AI winter".
Much more recently, a colleague of mine wanted to show some photos he'd taken of a huge peanut spill on a countryside road. A truck carrying a large amount of peanuts had apparently encountered some malfunction, dumped its cargo, and left it there for grabs. He tried searching his smartphone photo library for "peanuts", but no images materialized. I suggested to instead try searching for "pebbles" and, lo and behold, we were immediately met with pictures of a sea of peanuts.
The image recognition feature on smartphones is one of the things that now fall under the "AI" umbrella: a piece of software trained on a very large number of photos in order to classify and recognize what they depict. The problem is that even in a very large selection of photos, seas of peanuts are extremely rare. To find a photo of one, a human must step in and do something AI software is so far incapable of: knowing how the software was trained and use that knowledge creatively - such as coming up with the visual similarity between peanuts and pebbles. This practice is called prompt engineering.
Prompt engineering is not only a rather silly name, it's also a paradoxical practice in the world of AI. The whole selling point of the latest AI hype is to make computers behave more like humans. It's what end users expect, and it's what all the major players are using to drive the current hype. Type in (or speak!) a question in normal conversational English and get an answer back that reads (or speaks!) in a similar style. Ask it to find pictures of flowers, and pictures of flowers is what you get.
The need for prompt engineering, on the other hand, puts us back on square one of computer use: the pesky old conundrum of a human user having to think like a computer, instead of the other way around.
***
Is it lemons? Is it soap in the shape of lemons? Is it lemons
in a net for soap in the shape of lemons? The household robot
doesn't know. Therefore, it screams.
It's not that AI, in the broadest sense, isn't already useful. A lot has happened since the 1960s, especially regarding hardware. Faster and cheaper machines can run more complex software and process much bigger data sets. We're now at a point when machine translation is prevalent. It might not be perfect, but it's often good enough and - just like smartphone image search - often better than nothing.
Image recognition isn't necessarily something to scoff at either, when applied correctly. If it can help human doctors detect certain diseases with improved speed and accuracy, it's already a great tool. The cost of developing and running it is then also easily outweighed by saving lives. The problem is that public perception tends to treat such extremely narrow use cases a bit like it treats computers playing chess.
A modern chess engine can easily outplay even the top ranked chess players of the world. It can be useful for practice and even developing new styles of play, but using one in a chess tournament is considered cheating. Such use is considered cheating for the same reason it's also considered uninteresting: Humans want to watch human feats. To most people these days, a computer playing chess comes off as an extremely computery activity. Everyone understands that chess is a closed - albeit complex - system. Everyone also realizes that a modern computer can make deeper, faster and better predictions than any human is capable of. It isn't interesting, impressive or entertaining - at least not the same way a 12 year old human chess prodigy is.
A computer that can detect a certain type of disease is of course more interesting and beneficial than a highly competent chess engine, and is going to be accepted by the vast majority of humanity as something good. It's not cheating, it's helping. Yet, it's not much to hang a bunch of hype on: Like with a chess engine, or halfway decent machine translation, it's simply a computer finally doing one of the many things we've always been told they should be able to. A one trick pony, basically just another piece of medical software, more like Word or Excel than a thinking machine.
***
Thinking machines are, after all, not just what the words "artificial intelligence" mean to most of us. It's also what the big companies in the field are currently selling: Software that can't merely communicate in a human-like way, but also ostensibly act human-like - except smarter, more knowledgeable. Thus, the general expectation is that AI implies, at the very least, software that consistently and reliably outperforms a human expert at any task in any given field it claims to be proficient in. Failing that, it should at least be aware of its limits, letting the user know if a certain question can't be answered or a certain task can't be performed satisfactorily.
The problem is that so far, we've only managed the human-like communication part, and even that is still a bit iffy at times - ideally, it shouldn't require curious prompt engineering in order to produce high quality results. The other half remains riddled with ineptitudes, the biggest being so called hallucinations. Hallucinations are when a Large Language Model - currently the popular definition of an AI system - confidently presents falsehoods or utter confabulation as facts. LLMs do this because they cannot think, or know things, and thus cannot discern true from false, and thus can't stop themselves from blurting out stupid or downright dangerous answers. Whether or not this behavior can be completely mitigated or not is dubious at best: At their core, what LLMs do is string together words in a statistically highly probable manner. It's not just about "junk in, junk out" - which remains a problem because of the sheer size of the data sets used to train them - but also about completely eliminating the risk of anomalies, ensuring the model can somehow determine if it's generated one.
***
OpenAI's ChatGPT is one of the most well known LLM offerings right now, and the company itself is backed by some very wealthy investors, including Microsoft. They're also very good at creating hype. Though they've had their fair share of controversy, they're still treated amicably by the press, who dutifully help them feed said hype when launching a new version of their tech.
This hype initially made a lot of people very enthusiastic about LLMs - including yours truly. Some of this enthusiasm can be ascribed to misunderstanding the technology in question - but both academical communication and corporate marketing surrounding these products is to a large degree what's been feeding misunderstandings and unrealistically bolstering expectations.
The megacorps behind most of this tech, and a small but vocal group of so called tech bros, are very good at maintaining image. Minor improvements generate major excitement, while complete failures are swiftly forgotten. OpenAI recently demonstrated their newest ChatGPT, version 4o, talking to the user in a very human voice from an iPhone. Whether or not the model still hallucinates freely and confidently presents falsehoods as fact was, of course, not mentioned in the demo. The damn thing adviced some dude not to wear a silly hat to a job interview, so Shut Up and Sit Down: the Future is finally here!
Meanwhile, blatant failures such as Meta's Galactica, the science LLM that immediately after launch hallucinated its way out of any kind of usefulness, are quickly swept under the rug. The fact that such a model was launched at all hint at either a sunk cost fallacy in play - or just people believing their own hype. Neither option is a sign of sound business practices and surefire investment opportunities.
***
It seems that reality is finally catching up with the hype, and the enthusiasm is predictably starting to fade. More than one lawyer has used ChatGPT in their job only to end up in serious trouble, having to answer for erroneous citations and hallucinated court cases. The instinctive reaction might be to make fun of said lawyers, but in this case some reflection might be pertinent. Was it stupid of them to trust ChatGPT? Yes, of course. And who, if not a lawyer, should study the fine print of a product before using it? Still, this behavior reflects what a lot of people expect of AI: What's an LLM good for if it can't even get a simple case citation right? This feels like a basic minimum considering the hype. It doesn't even require reasoning or creativity, it simply requires correct regurgitation of well documented facts.
Even among tech-savvy people, the hype is - or at least was - strong. Not that long ago it was commonplace for conversations with intelligent, successful people working hands-on with IT to completely veer off into AI fantasy land. Wild ideas about how an LLM could supposedly extrapolate a successful corporate budget and strategy based on nothing but a handful of isolated KPIs apparently seemed completely reasonable. Or, based on the same handful of KPIs, an LLM could perhaps write an entire annual financial report, ready for print? Suggestions like these have become noticeably less prevalent in merely a year's time.
The truth is that as of yet, LLMs aren't even remotely close to this. In fact, they can't even reliably summarize a text, because they might miss a single piece of vital information hiding in there. Anything coming out of an LLM that's going to be used in any context of importance must be thoroughly double checked by a human. Congratulations: the manual workload now consists of scrutinizing not one, but two bodies of text!
Despite this, an LLM can, maybe, be somewhat useful some of the time. Take programming for example. We're still quite far from "no code" development, where an ideas man typing up a few requests in a prompt is all that's required for an AI to churn out a working piece of software. We're even quite far from reliable and consistent code generation even for rather simple cases.
However, for common tasks in common frameworks written in common languages, an LLM can produce boilerplate code which can then be corrected and expanded by a programmer. Or, for certain tasks, it can generate code in a language unfamiliar to an otherwise skilled developer, who can then test and correct it using some human know-how and creativity.
On the flip side, for example when working with a 20-odd year old legacy code base, an LLM is less useful. The same goes for reliably finding bugs, backtracking previous decisions or even understanding and modifying already existing code. Or how about motivating a decision - for example explaining why something can't be done to the human requesting it?
The same can be said about image generation, machine translation and even medical applications. For highly specific use cases, a human professional can be somewhat helped. In even more specific cases, a human could perhaps be entirely replaced - such as for churning out covers for dime-a-dozen sci-fi novels. In all other cases, it's little more than a fun novelty that will repeatedly and predictably make up nonsense, screw up basic human anatomy or mistranslate crucial, domain-specific words. We can, as of yet, not place our trust in a perfect and infallible machine.
This also applies to self-driving cars. Driverless vehicles in closed systems have been in use for a long time. The Copenhagen Metro, for example, has been in operation since 2002 - but like a chess engine, it isn't "AI": it's simply "automated". Currently available software may very well make human drivers both more comfortable and safe, but the hype has promised completely autonomous cars reliably zipping about in rush hour traffic.
Much like hallucination free LLMs, this has been "just around the corner" for quite some time now. In reality, the software is still unable to recognize the many types of confusing situations that appear in everyday traffic and, more importantly, lacks the ability to improvise accordingly. In its present state it can be helpful for certain tasks, but only under constant human supervision.
***
Recently, I wanted to change the start page in Microsoft's Edge browser from their bloated Bing monstrosity to a completely blank page. I searched Bing for an answer but couldn't find one. I then decided to try out the chatbot, as suggested to me by Bing itself. The result was utterly and unequivocally farcical: the bot simply answered with a somewhat rehashed version of the top search hit. When pointing out that the answer mentioned nonexistent menu options and settings, the bot simply apologized and presented a rephrased version of the next search hit, which was equally useless.
As a professional programmer with extensive knowledge about computers and software, I can understand why this happens. As an end user, it's both baffling and disappointing: Microsoft's own chatbot running on Microsoft's own site in Microsoft's own browser can't answer a simple question about how to configure that very same browser. It wasn't even "better than nothing": it was a worthless waste of time and effort, making "good enough" seem like a bad joke. If it didn't know, or if the setting doesn't exist, why didn't it say so? And if there is a setting, why couldn't it just fix it for me? Surely a Microsoft AI should know how to safely and correctly operate one of Microsoft's own flagship products.
Proponents of the current hype will of course say that problems like these can and will be corrected. With big enough data sets and extensive enough reinforcement training, hallucinations and wild goose chases will disappear. Or, at the very least, we'll somehow get models that can say "I don't know" instead of spewing out garbage.
Personally, I'm not convinced - but let's be generous and imagine it's achievable. What would that look like?
If public confidence in self-driving cars is ever going to match the hype, these cars must perform flawlessly. They can't just be as good as the average human driver. Their mistakes must be so rare and benign that they're completely statistically insignificant, and their performance must be reliable in freak weather conditions and on subpar roads. And even then, the problem of accountability must be resolved satisfactorily when it comes to things like insurance and court mandated victim recompense.
If we're going to be able to use LLMs to replace certain professions, they must at the very least match the average human, yielding consistent, reliable and reproducible results while making fewer and less costly mistakes. And, they should of course be capable of this without extensive and tedious prompt engineering. The question of responsibility and liability is a pressing one here, too.
***
A third category is one where chatbots, despite their flaws, are already replacing humans. Indeed, customer support seems like a perfect fit for AI: it has already, very aptly, been a dystopian nightmare for ages. Interactive voice response, rigid troubleshooting flowcharts, scripted replies, zero agency or lenience and abysmal working conditions. Who cares if the LLM fails to deliver? The prevalent notion already seems to be that if the complaint falls outside the top three, no procedure exists to handle it. The customer then becomes a sworn enemy - albeit an enemy legally required to keep paying for the duration of the contract.
Half-joking aside, will a "digital customer assistant" that sometimes barfs out false or even dangerous answers ensure smooth and profitable corporate operations? Is it cheap enough that the tradeoff, in the long run, is worth it compared to other tech and staffing options? And, most importantly, is crappy customer support really the product AI companies are selling to investors and consumers?
A shirt with no stripes, you say?
Even when it comes to something like LLM-assisted programming, where a highly skilled developer can maybe, sometimes, somewhat gain a performance boost, the most pertinent question isn't if it can be done at all - but rather if what can be done good enough can also be done profitably. The number of GPUs and the amount of increasingly expensive energy required remains as unclear as the time frame needed to accomplish it.
Still, the hypemeisters are unable to stop dropping thinly veiled hints about artificial general intelligence - "thinking machines" - from time to time. Any day now! And that last LLM that couldn't really do what we said it could? That wasn't real AI. This time though, it is. Honestly. Promise. Sort of. Thus, for every new LLM version, user disappointment with the AI hype seems to increase.
And let's not even get started on intellectual property in training sets, copyright and ownership of generated content, and liability for erroneous information or conduct. These are areas corporate lawyers just can't wait to sink their teeth into, given the opportunity. Who wouldn't want to get drawn into a big, juicy lawsuit with Disney or IBM over what constitutes fair use or patented code? Trust me, investors and execs live for that shit!
***
Things are looking rather bleak for the tech business as a whole right now. The economy no longer allows for zero interest loans or pouring endless streams of capital into vague promises of "real soon now". AI seems to be the last bastion of this practice: OpenAI, for example, were recently praised for their record growth in revenue and received substantial injections of capital.
But we can only pretend for so long that revenue right now says anything at all about profit in the future. There's still no indication of whether a reliable AI will cater to a broad enough customer base, be cheap enough to use and remain lucrative enough to stand on its own two proverbial robot feet.
I may, of course, be completely wrong. Perhaps we'll all soon be replaced by a handful of very small shell scripts interfacing with a distant AI's API. But, deservedly or not, it seems more likely to me that winter is coming.
What's Your Reaction?


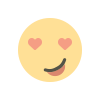
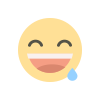
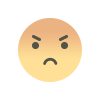
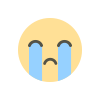
